An Yan, who received her doctorate degree in information science at the University of Washington (UW) earlier this year, and Bill Howe, UW Information School faculty member and West Hub co-principal investigator, recently presented EquiTensors: Learning Fair Integrations of Heterogeneous Urban Data at the 2021 International Conference on Data Management. Howe and Yan shared results from their studies on increasing fairness in urban data regarding mobility and transportation – as related to race, equity and diversity. Their paper suggested a unique machine learning concept they created called EquiTensors, an unsupervised approach to learn integrated and equitable data representations, that aids in fairness with urban mobility and transportation.
“Our literature review showed that disadvantaged groups of people experience inequitable public transportation access,” said Yan, who focused her doctoral research on fairness in machine learning, spatial-temporal prediction and urban mobility.
The Equitensors architecture was designed to learn fair, reusable, integrated representations of heterogeneous city data to improve performance of mobility prediction tasks while managing equity considerations. This approach helps decrease discriminatory effects inherent in public data while preserving utility.
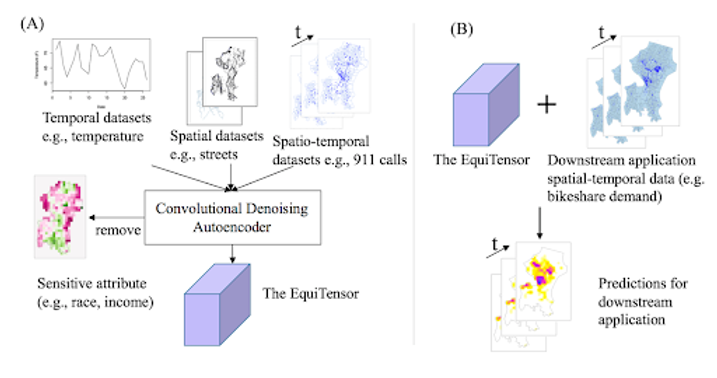
EquiTensor Architecture
(A) EquiTensor is in integrated representation from multiple urban data sources, with the sensitive attributes (e.g. race, gender) largely removed. (B) EquiTensor can be used in various urban mobility tasks to help improve prediction performance. Credit: An Yan and Bill Howe. “EquiTensors: Learning Fair Integrations of Heterogeneous Urban Data.” Proceedings of the 2021 International Conference on Management of Data. 2021.
Yan and Howe considered spatiotemporal patterns of public safety incident reports and stationless bikeshare demand. The learned Equitensors, when used in forecast models, showed reduced correlations with sensitive attributes (income and race) while maintaining accuracy. These results provided evidence for controlling bias in urban prediction applications that require integrating multiple sources of data.
“With EquiTensors, we first align source datasets to a consistent spatio-temporal domain, then describe a self-supervised model based on convolutional denoising autoencoders to learn shared representations,” explained Howe. “We extend this core integrative model with adaptive weighting to prevent certain datasets from dominating the signal and to combat discriminatory signals in the data, we use an adversarial model to ‘unlearn’ correlations with a sensitive attribute such as race or income – in this way, we teach the model to remove the effect of a sensitive attribute from the data.”
Howe and Yan conducted experiments with 23 input datasets on multiple mobility applications and showed that learned representations EquiTensors can simultaneously improve performance of downstream applications while mitigating discriminatory effects.
For more information about EquiTensors, please visit EquiTensors | Proceedings of the 2021 International Conference on Management of Data (acm.org).
This research is funded by the National Science Foundation Award 1934405.
About the West Big Data Innovation Hub:
The West Big Data Innovation Hub is one of four regional hubs funded by the National Science Foundation (NSF) to build and strengthen strategic partnerships across industry, academia, nonprofits, and government. The West Hub community aims to catalyze and scale data science for societal needs – connecting research, education, and practice in thematic areas such as natural resources and hazards, metro data science, health, and data-enabled discovery and learning. Coordinated by UC Berkeley’s Division of Computing, Data Science, and Society, the San Diego Supercomputer Center, and the University of Washington, the West Hub region includes contributors and data enthusiasts from Alaska, Arizona, California, Colorado, Hawaii, Idaho, Montana, Nevada, New Mexico, Oregon, Utah, Washington, Wyoming, and a global network of partners.
Related Links:
West Big Data Innovation Hub: westbigdatahub.org
National Science Foundation: www.nsf.gov/
The Big Data Innovation Hubs: bigdatahubs.org